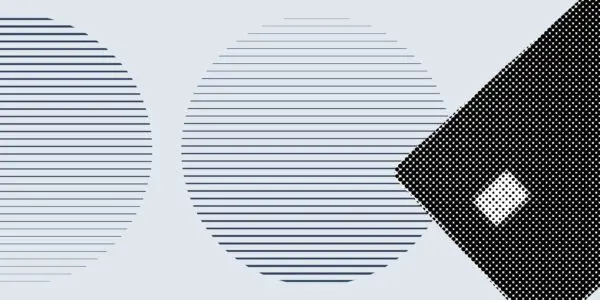
The role of technology and talent in transaction screening
Get actionable insights on optimizing screening processes to manamage financial crime risk.
Download your copyAs part of their regulatory compliance obligations, banks and other financial institutions (FIs) must develop and implement risk-based AML programs to counter the money laundering and terrorism financing threats they face. After performing risk assessments on their customers, firms should ensure suitable monitoring and screening measures are in place to alert them when high-risk customers engage in activity that could indicate money laundering.
However, an AML alert does not necessarily mean money laundering is taking place. The sensitivity of transaction monitoring and screening measures often means firms have to deal with false positive alerts when detecting genuine criminal activity. False positives constitute a significant proportion of the AML alerts generated by monitoring and screening measures, which means they can cause significant problems for firms by diminishing the speed and accuracy of their compliance processes.
A false positive occurs when AML software issues an alert for a transaction that seems suspicious but turns out to be legitimate. For example, a customer who makes several cash withdrawals from different bank branches on the same day may trigger an AML alert because these transactions are considered unusual – even if the customer has legitimate reasons for making them. Likewise, variations in global naming conventions can result in customers being incorrectly flagged during sanctions screening processes.
An FI’s AML compliance team would need to investigate both of these cases due to the alert – even if they are ultimately revealed to be false positives.
There are several reasons why false positives can happen, particularly if institutions have not taken all available steps to reduce their likelihood:
Get actionable insights on optimizing screening processes to manamage financial crime risk.
Download your copyFalse positives represent a challenge for FIs around the world for several reasons. They can:
While false positives can represent significant cost and efficiency drains, firms have little room to reduce the sensitivity of their screening and monitoring measures, given the financial, reputational, and even criminal penalties resulting from regulatory non-compliance. Finding a way to reduce false positives without compromising customer screening and transaction monitoring should be a priority for every compliance team.
While it is not possible to eliminate false positives, given the strict regulatory obligations firms have to meet, they can be reduced as much as possible with a few important measures:
Transaction monitoring and screening measures require firms to process and analyze a vast amount of data from various sources, often in an unstructured format. The more confusing the data collected as part of procedural compliance, the more challenging it is to discern false positives from true positive AML alerts.
By carefully considering the data capture process and structuring that data clearly once acquired, firms can improve their false positive rate. Practically, one example would be organizing names into title, first name, and surname rather than listing each name as a single data point. The latter approach can create administrative ambiguity and hinder compliance teams’ attempts to resolve customer identities after AML alerts. To further clean data, duplicated or irrelevant data points should be removed, errors identified and corrected, and any missing data addressed.
Criminals may seek to change their names or move between countries to thwart AML controls. With that in mind, while the quantity of AML data captured is important to building an accurate customer profile, the data’s relevance and recency are crucial to the verification process. For example, a false positive might be created if a firm fails to update a customer’s change of name or residence from a high-risk jurisdiction to a low-risk jurisdiction. The ability to update data in as close to real-time as possible represents a significant advantage to firms here.
As with other elements of AML compliance programs, a risk-based approach is crucial in reducing false positives. Taking a blanket or one-size-fits-all approach to screening and monitoring is highly inefficient and likely to inflate false positive rates through unnecessarily broad or undeveloped rules used to identify suspicious transactions. Clear risk parameters based on contemporary money laundering typologies should be used while proceeding from an initial business-wide risk assessment. Rules should also be formed specifically with a given institution’s risk profile, regulatory requirements, and services or products in mind.
An AML compliance program should evolve according to new regulations or the discovery of new criminal methods, not be a simple exercise in checking boxes. Accordingly, firms should conduct ongoing reviews of their screening and monitoring measures to ensure their continued accuracy. As regulations change and new financial crime patterns replace old ones, firms should ensure their place on the leading edge of AML/CFT and maximize their efficiency by adapting their compliance measures.
Smart technology is a crucial component of the modern financial compliance process. With the benefit of integrated artificial intelligence (AI) and machine learning models, firms can increase the efficiency and accuracy of their AML programs, building richer and more databases for use in risk assessments.
While AI and machine learning models shouldn’t be seen as a replacement for human expertise within the AML process, they can help compliance teams address false positive alerts in a more structured and efficient way. Optimizing AI and machine learning integration of AI should become a foundational part of the AML process for financial institutions and an essential step towards reducing a firm’s false positive rate.
ComplyAdvantage’s AI-powered matching algorithms screen new customers for sanctions, watchlists, adverse media, enforcement data, and PEP and RCA lists, drawing on dynamically updated databases to minimize false positives caused by inaccurate data. Our intuitive screening processes are also adapted to global customer naming conventions that could otherwise generate false positive hits on sanctions lists. At the same time, our ML models enable firms to perform semantic and statistical analyses on new alerts, quickly identifying duplicate or redundant data that might be creating the appearance of suspicious behavior.
Our combination of consolidated data, real-time updates, and smart insights driven by natural language processing empowers FIs to make informed decisions and lower their false positive rate.
ComplyAdvantage’s transaction monitoring solution draws on an extensive library of red flags and risk scenarios, with our AI capability filling in the gaps where no known rules exist.
Get a demoOriginally published 16 October 2020, updated 28 August 2024
Disclaimer: This is for general information only. The information presented does not constitute legal advice. ComplyAdvantage accepts no responsibility for any information contained herein and disclaims and excludes any liability in respect of the contents or for action taken based on this information.
Copyright © 2025 IVXS UK Limited (trading as ComplyAdvantage).